This post is my response to Ted Chiang’s recent essay in which he argued that AI isn’t going to make art. My thesis is in two parts: (1) I agree with the core thrust of Chiang’s argument that AI-generated art lacks “meaning” relative to human-created art, at least for the time being; (2) I also think that as a practical matter, generative AI already is and will continue to be a useful tool for humans to create art, and trying to insist on not calling such creations “art” is a losing battle.
Let’s start with a basic premise: deflationary theories of “art” are no good. Some people claim that art is just “whatever anyone says is art.” To me this claim belongs in the same family of pseudo-insights as “truth is just whatever anyone feels like is the truth.” Yes, there is some subjectivity in both truth and art, but to deflate it entirely to “whatever anyone says”, and to claim that there is nothing more to say on the matter, is dishonest. If “art” and “not-art” were entirely made up and arbitrary distinctions—and by extension “good art” and “not-good art”—then we would never have had awards for art to begin with, we could never have the notion of “classics”, of reviews and ratings and critical acclaim, we would find it utterly impossible to converge on these things; galleries would never get up and running in the first place because it’s simply too subjective to figure out what to display. If “art” and “the goodness of art” was a totally arbitrary category, we would never talk about “the best art”; having awards for “the best art” would seem as absurd as awards for “the best color” or “the best childhood memory” or “the best dreams” or “the best morning routine.” All of these things—dreams, memories, morning routines—are things we talk about and care lots about, and yet we don’t have awards for them, because it is impossible to converge on a globally shared notion of “better” and “worse”—it is actually too subjective. That is not the case with art.
What people are really doing when they claim that “anything can be art” is synonymizing “art” with “beauty” or even “pleasure.” Art is just anything that looks nice or feels nice. I agree with Erik Hoel that this is overly reductive: the whole point of a word is to differentiate one thing from another, and if “beauty” is all that makes art, why not discard the term “art” entirely? A waterfall is beautiful, and so is the night sky, but neither of them are rightly considered “art.” In my view there was no art on this planet until humans (and perhaps some non-human ancestors) came along and created it. Art requires some degree of intentionality: it’s the result of someone trying to express something. (That “someone” need not necessarily be a biological human—but they do need to be an agent with the capacity to interact with and represent the world.)
Why does it matter whether a human (or some other agent) created a work of art? Isn’t the beauty and meaning of the art inherent to the work itself? In his essay critiquing AI art, Chiang gives a number of examples for why this is generally not true—we generally do care about the intentionality behind what we consume, rather than only caring about the pure information content. You would be more excited to read a postcard that your friend wrote to you than a postcard that someone else wrote for their own friend, even if the contents of the two postcards are roughly the same (e.g. “miss you and hope you’re doing well”). Similarly, we care about whether we’re watching Elvis Presley live, or just a guy who looks and sings almost exactly like Elvis Presley. We care not just about the raw sensory stimulus, but about what lies behind it (both in physical space, and also in time). We would pay more to see the Mona Lisa than a replica of it; we care more to see Taylor Swift in person rather than a Taylor Swift impersonator who has practiced how to look and behave exactly like her. It’s the whole causal history of interactions leading to the current moment that makes a given communication or work of art meaningful to us.
You might dismiss this as pure sentimentality. But what I would like to posit is that this is how meaning in general works: meaning is created by a process of interaction, rather than being inherent to the information content of the message. I will try to show that this is true for both senses of the word “meaning”: there’s semantic meaning (like when someone says “what does the word bequeathment mean?”), and there’s also existential meaning (like when someone says “that was a super meaningful experience for me”). Both kinds of meaning arise from interaction rather than being purely a subjective feeling or being inherent to the content.
The particular view of meaning I’d like to put forth has a pretty deep influence on the way you experience language and thought as a whole, and it also happens to be one of the theoretical foundations for the current generation of language models, so it’s worth explaining in detail. Of course, “the problem of meaning” is something philosophers have grappled with for many decades, and will be hard to completely settle in the course of a few paragraphs—but that is what I’m going to attempt to do, before getting back to talking about AI.
Meaning is interactive
Let’s talk about an example that will seem silly at first but will hopefully illustrate my point.1 Imagine a shepherd needs to keep track of his sheep—he has a bunch of sheep in a big pen, and every day he lets them out to roam freely in the field, and he needs to make sure all of them have returned at day’s end. To keep track of the number of sheep that have left his pen, he devises a simple method using a bucket and a bunch of pebbles. The bucket starts empty, and every time a sheep leaves the pen, the shepherd drops a pebble into the bucket. Every time any of the sheep re-enters the pen, the shepherd takes a pebble out of the bucket. In this way, the bucket of pebbles tells the shepherd exactly how many sheep are currently outside of the pen, so he knows at the end of the day if any of them are missing. Thus a notion of “meaning” is born: the meaning of the pebbles is the number of sheep who have left.
And now the question is: how did this meaning come about? Do the pebbles “reflect” the sheep in some physical sense? Do they have some magical counting property? No. It’s the shepherd’s actions that give the pebbles meaning. If the shepherd stops keeping count and throws away the pebbles, they no longer have any meaningful relationship to the sheep. If it just so happens that someone else walks by with a bucket of two pebbles, and at that moment there happen to be two sheep outside of the pen, we wouldn’t say that the second bucket also represents the number of sheep outside the pen—it only does so by coincidence.
The point is: meaning arises from intentional activity. It’s the shepherd’s ongoing commitment to keep the pebbles “in line” with the state of the world that gives them meaning. Meaning a process rather than a specific state at a specific time—or as David Chapman puts it, it’s not strictly subjective nor strictly objective, but interactive.
Now, the rather disorienting part of this is that you can apply this point to more than just pebbles in a bucket—it applies to language in general, and it even applies to our thoughts. When you write down on a piece of paper (or have the thought in your head), “get a carton of eggs and a gallon of milk tomorrow”, what gives that utterance its meaning is nowhere to be found in the shape of the symbols themselves. The symbols on their own are just a bunch of squiggles—there is nothing in the structure of the squiggles making up “egg” that corresponds to actual physical eggs. The symbols derive their meaning from the way they’re used in a specific cultural context (i.e. the English-speaking world). The meaning comes from the way that we, collectively, use those words.
We all have a very deeply held intuition that the abstract thoughts in our head are intrinsically meaningful and simply refer to things in the world, purely by virtue of their internal structure. But this is a mistaken intuition, and here’s one of the more obvious reasons why: it’s possible to be totally convinced that your thoughts/utterances are meaningful, even while they are completely devoid of meaning. This is one of the failure modes of specific kinds of philosophy, and is something anthropologist Dan Sperber talks about in his paper The Guru Effect. Sperber lists a number of examples of famous philosophers with devoted followers, who tended to make statements that were at best unclear, and at worst completely nonsensical. Consider, for example, this paragraph by postmodern philosopher Jacques Derrida:
“If différance
is(and I also cross out the ‘is’) what makes possible the presentation of the being-present, it is never presented as such. It is never offered to the present. Or to anyone. Reserving itself, not exposing itself, in regular fashion it exceeds the order of truth at a certain precise point, but without dissimulating itself as something, as a mysterious being, in the occult of a nonknowledge or in a hole with indeterminable borders (for example, in a topology of castration).”
Do you understand what Derrida means here? Does he mean anything? I’m certain that he felt like he meant something by this statement, and maybe it does actually mean something; and yet it’s equally plausible to me that it has as much meaning as the babbling of an infant, as much semantic content as me saying “Gloobly flurp zibbit mox frandled squerking noobles plinky doo.”2 How do we figure out whether Derrida’s statement falls under the category of “meaningful” or “meaningless”? My point is that we can’t answer this question by parsing, in ever more detail, the specific words that he uttered; we answer it by stepping back and looking at how his words were used in the world, how they influenced his and his readers’ behavior. As Wittgenstein put it: meaning is use.
Now, everything I’ve said so far applies to semantic meaning, but what about the second kind of meaning—the existential kind? My sense is that interaction is also a requirement for this kind of meaning. When you say an experience was very “meaningful” for you, what that generally means is that it has a large number of causal connections to the way you see the world and your future actions. To say that an experience—or a work of art—is “meaningful,” is more than to merely say that it’s intense or pleasurable; it’s to say that the experience or artwork has tangible implications for your experience and actions. Again: meaning is use!
Back to generative AI
My point with elucidating all of this is that I agree with Chiang’s general stance on meaning. At first glance, when you chat with ChatGPT you might say: clearly everything it’s saying is meaningful. When it says “the capital of France is Paris,” it’s talking about actual things in the world and it has some understanding of what it’s talking about. It can reason and think in much the same way a human can.
But Chiang makes an interesting observation:
It is very easy to get ChatGPT to emit a series of words such as “I am happy to see you.” There are many things we don’t understand about how large language models work, but one thing we can be sure of is that ChatGPT is not happy to see you. A dog can communicate that it is happy to see you, and so can a prelinguistic child, even though both lack the capability to use words. ChatGPT feels nothing and desires nothing, and this lack of intention is why ChatGPT is not actually using language. What makes the words “I’m happy to see you” a linguistic utterance is not that the sequence of text tokens that it is made up of are well formed; what makes it a linguistic utterance is the intention to communicate something.
“ChatGPT is not using language” is definitely a provocative way to put it, but Chiang has a point. Language models are notorious for their tendency to hallucinate—like when GPT-3 said things like “Egypt was transported for the second time across the Golden Gate Bridge on October 13, 2017”—which might make us wonder whether the model really “means” what it says, or whether there’s some deep sense in which is “untethered” from reality and lacking genuine understanding of its utterances. You wonder if ChatGPT is, first and foremost, a bullshitter.
This would align with the notion of meaning we just described: if meaning is born of interaction with the world, language models evoke very little of it because they do very little interacting with the world. Yes, they go through a long training phase in which they ingest unfathomable amounts of text (and images, etc for multimodal models), and they do interact with humans through text boxes, but they rarely use language in the way that humans do. This could be one reason why they need so much more text than we do in order to be able to speak like us: whereas a baby can learn what a “cat” is by having an adult simply point to one and say, “that’s a cat”, there is no such real-world “pointing” available for an LLM. The LLM can only learn what a cat is by virtue of the way that the word “cat” appears next to other words. The LLM never learns how language is used in the world—only the internal structure of language itself.
Here’s a concrete, present-day example of a language model not really “meaning what it says”: if you ask ChatGPT or Claude to play a game of twenty questions, they will happily oblige, claiming that “I have an object in mind” that you are supposed to guess by asking it yes/no questions. The problem is, it’s impossible for the language model to actually “keep an object in mind” across a multi-turn conversation, without writing out that object into the chat itself, because current-generation LLMs are stateless: the only information that persists between messages is the text content of the chat itself. Of course, when you play the game, it sure seems like the LLM is keeping an object in mind, because it does keep all of its answers consistent with past responses. But the reality is probably more that the LLM “converges” on an object randomly over the course of the conversation.3
So: LLMs have a lot of difficulty with genuine meaning-making because they are not autonomous agents embedded in the world. But still, these models have gotten way farther than anyone could have reasonably expected, simply by looking at the internal relationships of words and images, rather than how any of these things are actually “used in the world.” This is why, despite all the differences between humans and generative AI that I’ve outlined—all the apparent limitations of language models—I have difficulty asserting that they are still just “stochastic parrots,” that they truly understand nothing of what they are talking about.
Instead, I think it’s better to think of “capacity for meaning” as a spectrum. A given person, or AI model, can have a higher or lower tendency to utter meaningful versus meaningless phrases, depending on their age, personality, and what they’re held accountable to. Infants babble nonsensically, and even teenagers and young adults are liable to parroting phrases whose meaning they don’t fully grasp until they’re much older. We see this contrast again and again in different kinds of people. Some politicians speak candidly and concretely, while other politicians can’t stop spewing verbal salad. The best business leaders speak in a way that genuinely motivates employees, while mediocre managers can’t help but put out bland corporate-speak. There are genuine spiritual leaders who provoke insight and discernment in their followers, and there are pseudo-enlightened gurus and intellectuals who utter fashionable nonsense. As humans, we are capable of not only being wrong, but being so confused in our statements that we are not even wrong. Generative AI, by virtue of being an unconscious,4 disembodied thinking machine, has even greater capacity for nonsense than us, because it has the least “grounding in reality.” So we should treat its utterances with a cautious distance, remembering that, just like the devout followers of fake spiritual gurus, an utterance or a work of art from AI can feel meaningful to us and still be completely meaningless.
Which brings us back to art. Just as these models have a high capacity for bullshit in language, they also have a high capacity for bullshit in art. But this is where I depart from Chiang: this doesn’t mean that they are incapable of participating in a meaningful act of communication or expression. It is precisely their use by humans (i.e. agents with intentions that are capable of representing the world) that imbues their creations with meaning.5
Art as it’s used, and the semantic apocalypse
If we put aside philosophical speculation about meaning, what is art, as a practical matter? Art is stuff we put in galleries for public consumption, or display in our bedrooms to make them feel like home; it’s stuff we use as our desktop and phone backgrounds; it’s stuff we gift our friends; it’s stuff we turn to when we feel lost or when we seek consolation. Art is also stuff we create in order to express ourselves; it’s an activity we engage in to build a sense of joy and mastery. It’s fine for AI to serve some of these functions, or to support us in fulfilling some of them. But I don’t want a world where AI art serves all of these functions, where it has replaced human-made art everywhere.
And yet, I have trouble imagining that ever happening. Despite the fact that today we have photography and digital art, people still paint things on actual physical canvases with actual physical paint. Photography and digital illustration never “automated” painting; likewise I don’t think it’s correct to think of AI as “automating” art. As Chiang points out, art is something that necessarily involves creative choices; the whole point is to take responsibility for as many of those choice as you feasibly can, rather than deferring all of them to an automation. In this view, generative AI becomes a tool just like cameras and digital illustration software.
Chiang claims that generative AI won’t become a tool for artists because the companies behind these models won’t tailor them towards people who want to put a lot of effort into the creative choices involved in making true art. This seems like a failure of imagination to me. Chiang’s own article describes the case of film director Bennett Miller, who exhibited AI-generated art at a gallery after generating over a hundred thousand images and whittling them down to just twenty. But he asserts that his won’t happen generally—that AI companies won’t make models that allow you to “enter tens of thousands of words into a text box to enable extremely fine-grained control over the image you’re producing.” And yet, someone commented on Chiang’s piece claiming that they’re already doing exactly that. As much as there are market incentives that might push these tools toward convenience over control, there will be others who seek out that control and build it for themselves.
My sense is that the most vocal critics of AI art are worried about something like a semantic apocalypse. That by virtue of AI-generated content being untethered from actual physical embodiment and interaction with the world, our culture will devolve into a shadow of itself, full of replicas and lookalikes, devoid of real meaning. The concern is that this transformation will happen slowly—too imperceptible to notice day by day, but totally unmistakable in retrospect. Just as LLMs can collapse into meaninglessness when recursively trained on their own outputs, the fear is that our culture will stagnate and ultimately devolve, and will look much like the Facebook feeds that are increasingly full of eery, AI-generated crap.
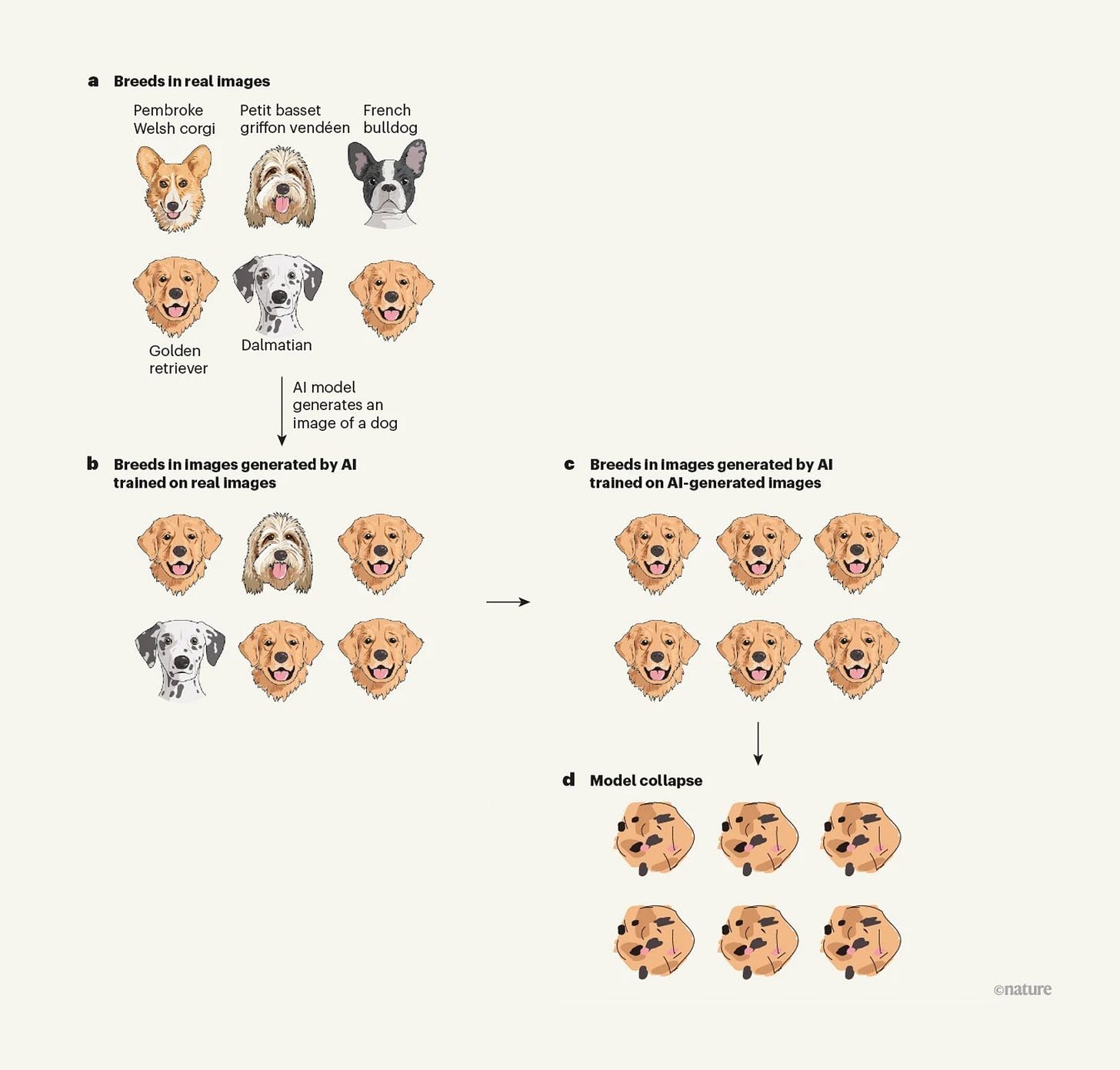
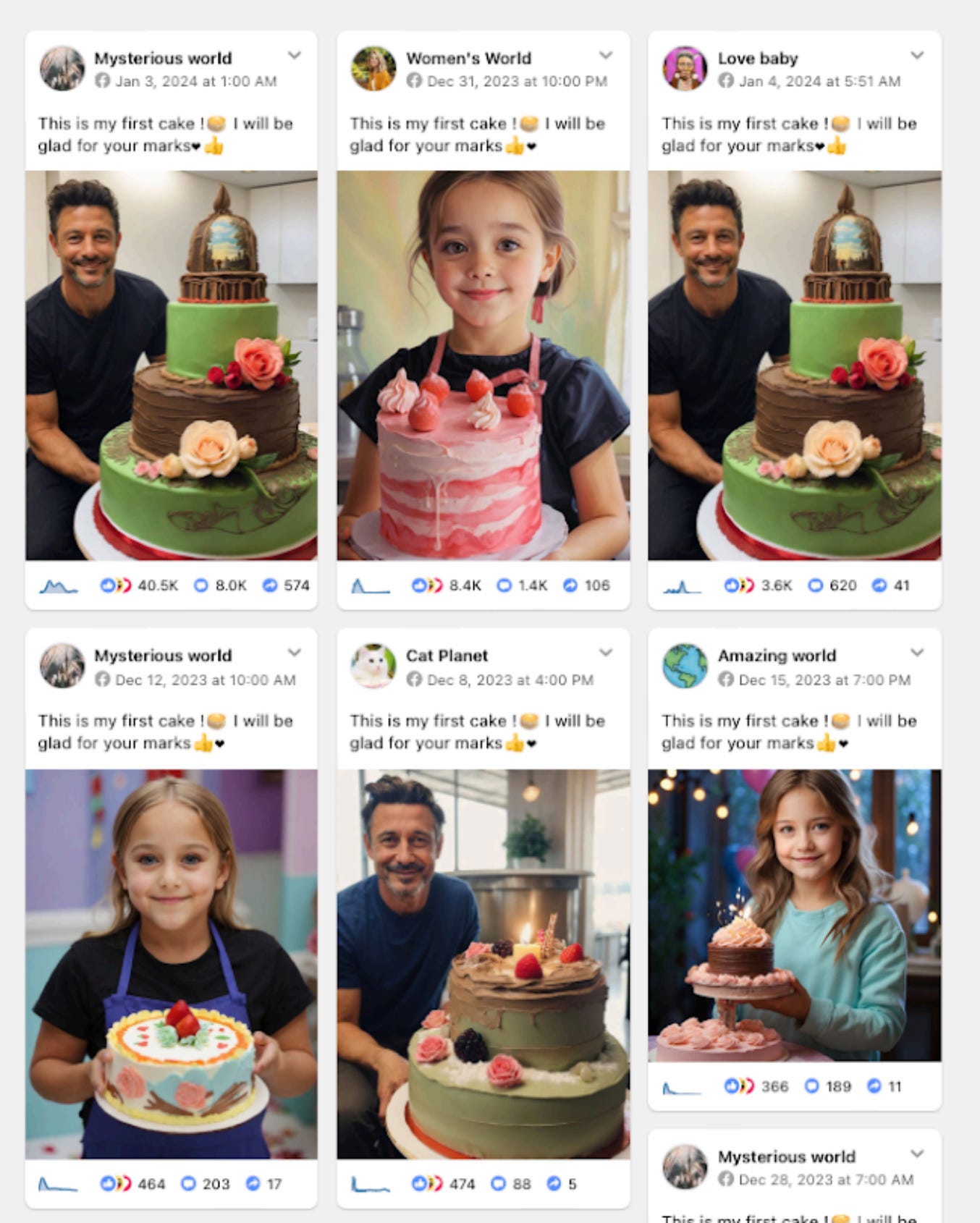
A future like this seems deeply disturbing—it’s a Brave New World saturated by superstimuli. Like male beetles that mate with beer bottles because the bottle looks more like female beetles than actual living females, we might become enamored by the artificial and disengaged from our actual lives. As Vivid Void puts it, we might build companions that “start as an AI friend to share jokes with and end as an LLM trained on your ex-wife who left you, or your deceased father, or the best friend who cancer took too soon.” We might lose our capacity to come face to face with grief, and loneliness, and all the other ills of real life, numbing ourselves instead with a pale substitute for real connection.
But humans are not beetles. I believe in the inextinguishable wisdom of the human spirit, in our desire for truth and genuine meaning. To the extent that AI is incapable of meaning-making, the most discerning among us will be capable of recognizing it as such. As humans, we can tell that superstimuli are actually understimulating—that’s why we decry them, because we can tell that something is missing. It’s why people choose to step away from their phones and social media, why “touching grass” is a meme, why we create minimalist computers that prioritize the user’s wellbeing rather than exploiting their compulsions. We won’t prevent a semantic apocalypse by insisting on particular definitions of words; we will do it by trying as hard as possible to create genuine meaning. The wisest among us will continue to do this, and they will inspire others to follow suit. It will always be true that individual humans can be fooled by a false idol, and sometimes even entire groups can be lured in by the simulacrum of meaning. But humanity as a whole will never be.
Thanks to Arkady and Ben for comments on earlier drafts.
This story is adapted from David Chapman’s parable of the pebbles, which I recommend reading in full.
Postmodern philosophers are generally criticized for this tendency to say a lot of things that don’t really mean anything; I think this criticism is sometimes fair and sometimes not. For an example of the latter, see this thread by Sasha Chapin explaining how Foucault, despite being very difficult to understand, was still extremely insightful.
Incidentally, LLMs also have trouble with the reverse of this game—where you have an object in mind and the language model is supposed to guess what the object is. They seem to struggle much more than humans at this, see e.g. these threads. They sometimes succeed at guessing, but often get stuck in long ruts because they can’t help but anchor on earlier questions (see this chat for an example; I’ve found the same pattern with Claude). My sense is that this is also a result of their lack of embedded interaction with the world, which generates a very hazy world model. As with past limitations though, it could also be that this is just a matter of better prompting and more scale.
Whether LLMs are conscious is a subject of debate for some, but my intuition is that they are not conscious, or that if they are, it is an extremely alien kind of consciousness which, again, doesn’t give them the kind of understanding of the world that we have. See some questions I’ve been pondering about AI consciousness.
An alternative approach here is to give the AI itself agency, a capacity to sense the world, an embodiment and distinct ongoing perspective and remembered life history—but as far as I know we aren’t doing this with these models yet, and I’m not sure they would be capable of being adapted to that purpose in their current form.
Such an intricate topic so clearly articulated! Loved reading this.
Fascinating piece